Health and Behavior
DEEP LEARNING FOR BIOMEDICAL HEALTH DATA
REU Scholar: Nidhi Begur
REU Scholar Home Institution: Florida Atlantic University High School
REU Scholar: Annelies Verbist
REU Scholar Home Institution: University of Edinburgh
REU Mentor: Behnaz Ghoraani, Ph.D.
PROJECT
Human activity recognition (HAR) in individuals with Parkinson's disease (PD) is a growing field in which artificial intelligence, in conjunction with wearable sensors, is implemented to aid in healthcare quality. Currently, the application of traditional machine learning techniques utilizing time-series sensor data is constrained by the sparse availability and the complex nature of the data. This led to the exploration of adopting a recent advancement in self-supervised contrastive learning, Simple Framework for Contrastive Learning (SimCLR). Contrastive learning is a technique in which we maximize the similarity between 'positive pairs', data of the same type, and maximize the difference between 'negative pairs' to learn to extract valuable features from the data. SimCLR creates its positive pairs through data transformations to extract these features. The evaluation of 64 unique combinations of transformations was utilized to assess the SimCLR models' performance in recognizing daily living activities in PD individuals. Compared to the traditional, state-of-the-art fully-supervised model, initial results suggest significant evidence that using SimCLR is more efficient in identifying these activities. In particular, SimCLR models with specific combinations of transformations demonstrated, on average, an improvement in performance over the fully-supervised model when tested on individuals with different degrees of disease progression. Thus, SimCLR should be continuously (can be further) explored to monitor patients' state of well-being effectively and aid healthcare professionals in identifying medication effectiveness.
REU Scholar: Brennen Farrell
REU Scholar Home Institution: North Carolina State University at Raleigh
REU Scholar: Julia Horn
REU Scholar Home Institution: Cornell University
REU Mentor: Behnaz Ghoraani, Ph.D.
Project: Detecting Alzheimer’s disease with Accessible Pose Analysis Methods
PROJECT
Alzheimer’s disease is a disorder that causes degradation and death of neurons, with more than 6 million cases, in the US alone. As this disorder is incurable, early detection is vital in the treatment of this disorder. This project seeks to aid in the detection of Alzheimer’s by testing if open source pose analysis methods are able to work with machine learning methods to create an accessible method of Alzheimer’s detection. After selecting AlphaPose and OpenPose as the pose estimation methods to be used, each method was on a dataset which contained both individuals with and without Alzheimer’s. Coordinate points were extracted as these pose estimation methods were run and features were calculated from these points. Significant features were then determined and ran into a machine learning model to detect whether patients had or did not have Alzheiemer’s. The combination of these pose estimation methods and the machine learning consistently performed at around a 90% accuracy, which was on par with or sometimes better than other methods such as the Microsoft Kinect Camera. Thus, it was concluded that these pose estimation methods were a valid method of detecting the presence of Alzheimer’s disease in patients.
Click here for pdf presentation
Click here to watch video presentation
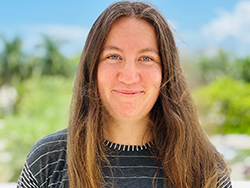